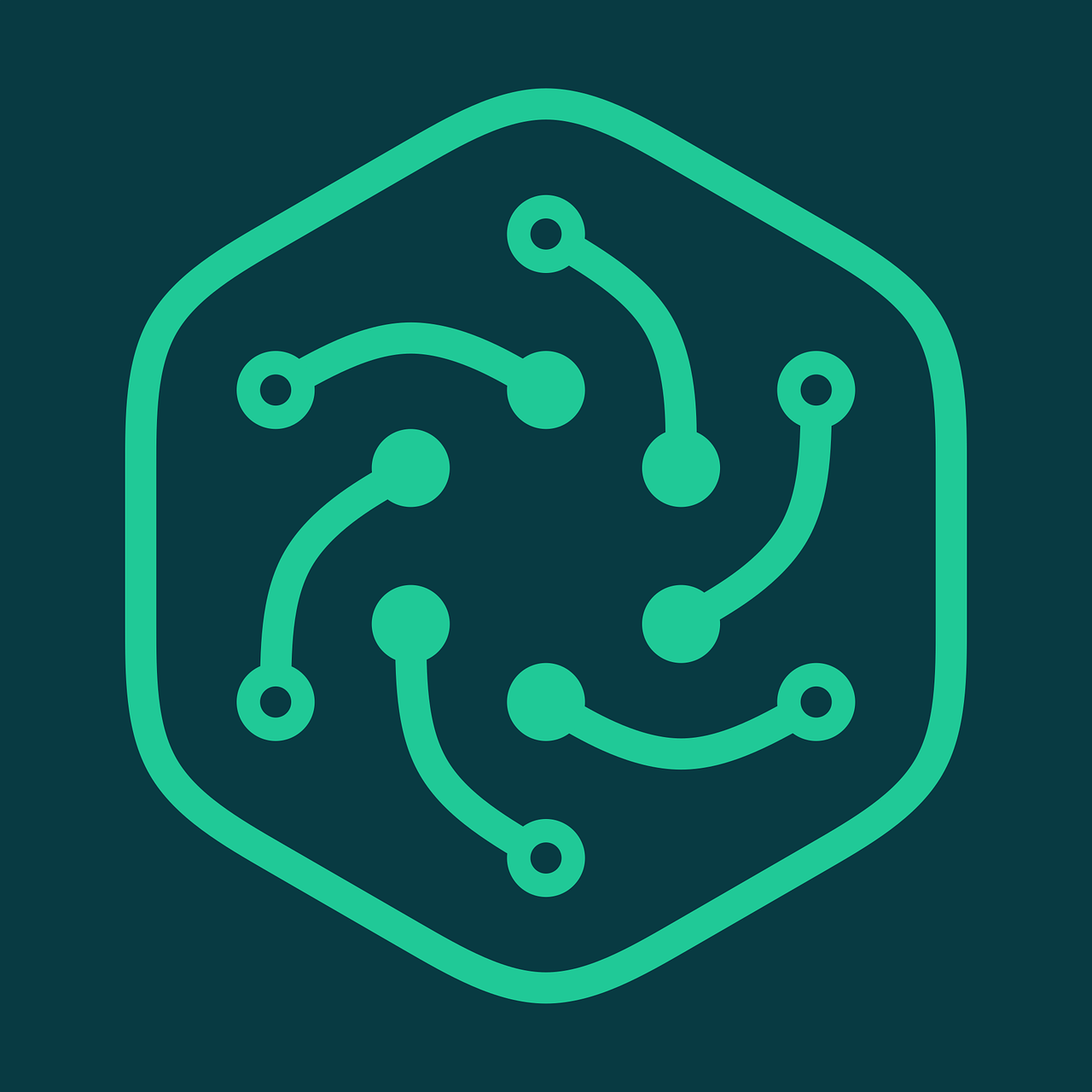
Introduction: Unveiling the Hidden Math behind AI Magic
Have you ever marveled at how your social media feed magically understands your preferences, or how an app can transform your selfies into artistic masterpieces? The digital world around us is teeming with invisible architects—Generative Adversarial Networks (GANs)—that shape the AI-driven experiences we encounter every day.
These innovative systems have taken the realm of artificial intelligence by storm, revolutionizing the way we perceive creativity. As we traverse the path of their evolution, we’ll uncover the stories behind the scenes, where ingenious minds sculpted the very essence of AI artistry.
The Birth of GANs: Crafting Reality Through Rivalry (2014)
A transformative event occurred in 2014 that would forever alter the course of we interact with computers. This groundbreaking moment marked the emergence of Generative Adversarial Networks (GANs), a brainchild of the brilliant mind of Ian Goodfellow and his visionary collaborators. GANs introduced a paradigm shift by unleashing the dynamic interplay between a generator and a discriminator—two neural networks locked in a symbiotic adversarial relationship.
The inception of GANs heralded a new era of AI-powered creativity. The generator’s task was to conjure content—ranging from images and texts to music—that would be assessed by the discriminator. The discriminator, in turn, assessed the authenticity of the content, distinguishing between real and AI-generated creations. This intricate dance between creation and critique forged the essence of GANs, embodying the spirit of artistic rivalry between algorithms.
GANs and Art: The Canvas of Creativity (2015)
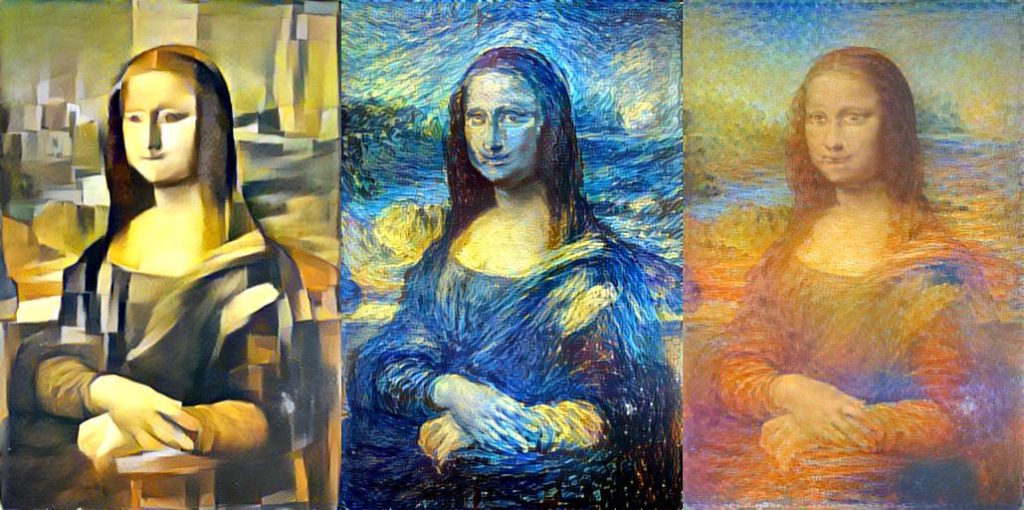
Imagine transferring the style of a Van Gogh painting onto a modern photograph. In 2015, Leon Gatys and his team unleashed the artistic prowess of GANs with Neural Style Transfer. This marriage of AI and art sparked a revolution where algorithms emulated the brushstrokes of the masters. Suddenly, the digital realm became a canvas, allowing artists to paint with pixels and programmers to dabble in artistic innovation. GANs emerged as the digital artisans of our era, bridging the gap between the past and the present.
From Pixels to Prose: The Textual Transformation (2017)
As GANs conquered images, they set their sights on the world of language. In 2017, Alec Radford and his colleagues at OpenAI turned GANs into storytellers. The once-restricted realm of human expression expanded as these networks weaved narratives, penned poetry, and even drafted code snippets. This transformation propelled GANs into the realm of creativity beyond vision, allowing them to navigate the intricate nuances of human language.
Progressive GANs: Realism and Video(2017)
The quest for higher resolution imagery led Tero Karras and his collaborators to introduce Progressive GANs in 2017. This technique allowed GANs to gradually enhance resolution, yielding images of unparalleled realism. From intricate facial details to sweeping landscapes, Progressive GANs breathed life into pixels, redefining the possibilities of computer-generated imagery. This also lead to the beginning of Video style transfer.
GANs’ Healing Touch: Medicine Meets AI (2018)
In the corridors of medicine, GANs assumed a new role: that of healers. Kang Zhang and his team harnessed their potential to refine medical images in 2018. These enhanced diagnostics promised precision and accuracy, potentially transforming patient outcomes. GANs became silent allies in the realm of healthcare, a testament to their versatile prowess.
Unveiling BigGAN: Embracing Scale and Detail (2018)
The world marveled at the audacious scope of GANs when Andrew Brock and his team introduced BigGAN in 2018. This monumental leap allowed GANs to generate high-quality images at an unprecedented scale. The digital world now teemed with complexity and clarity, as GANs demonstrated their ability to transform imagination into high-resolution reality.
Ethics Unveiled: The Deepfake Conundrum (2019)
In 2019, Hao Li and his team delved into the realm of deepfakes, harnessing the power of GANs to manipulate faces and voices with astonishing accuracy. This significant breakthrough raised critical ethical concerns, triggering widespread debates about the very nature of truth and authenticity in an increasingly digital landscape. As deepfakes gained prominence, their ability to blur the lines between reality and manipulation became glaringly apparent. Consequently, these developments sparked conversations about the ethical implications of AI’s creative capabilities.
The rise of deepfakes underscored the pressing need to address the potential risks of misinformation and deception. Policymakers, experts, and technologists alike urgently sought effective measures to identify and counter the propagation of falsified content. We are still in the middle of this issue
The Future Unfolds: GANs on the Horizon
GANs despite being more of a well known term is still a new and evolving method of Machine learning, from deepfakes to your tik tok filters or generate fully textured 3d models!
As GANs continue to evolve, they transcend industries, shaping the future of creativity and innovation. From generating music to crafting architectural marvels, GANs remain boundlessly inventive. Pioneers across fields embark on uncharted journeys, guided by GANs’ capacity to redefine the boundaries of creative expression.
Key Terms
- Generative Adversarial Networks (GANs): A class of machine learning models consisting of two neural networks, the generator and the discriminator, engaged in an adversarial training process.
- Generator: The neural network responsible for producing synthetic data, such as images, based on random noise or a latent input.
- Discriminator: The neural network tasked with distinguishing between real data (e.g., actual images) and data generated by the generator.
- Adversarial Training: The process where the generator and discriminator networks are pitted against each other in a continuous feedback loop, aiming to improve their respective performances.
- Adversarial Loss: The loss function that quantifies the discriminator’s ability to correctly classify real and fake data, guiding the generator to produce more authentic content.
- Latent Space: The multidimensional space where the generator operates, mapping random noise or input to meaningful output data.
- Mode Collapse: A phenomenon in which the generator produces a limited range of outputs, failing to capture the diversity of the underlying data distribution.
- Vanishing Gradient Problem: A challenge where the gradient updates during training become very small, leading to slow convergence or no learning in GANs.
- Conditional GAN (cGAN): A variant of GANs where both the generator and discriminator receive additional information (conditions) to improve control over generated data.
- CycleGAN: A type of GAN used for image-to-image translation tasks, such as converting images from one domain (e.g., horses) to another (e.g., zebras).
- StyleGAN: An advanced GAN architecture that allows control over the style and attributes of generated images, enabling the creation of highly realistic and customizable visuals.
- GAN Inversion: The process of reverse-engineering a generator to find a latent input that generates a specific desired output.
- ArtGAN: A GAN specifically designed for generating artistic creations, such as paintings or drawings.
- GAN Zoo: A colloquial term for the wide variety of GAN architectures and their applications, often emphasizing the growing diversity and complexity of GAN models.